AI Text Scores
Launch of The Text Score Dataset 1.0 by Jennifer Walshe, Darmstadt 3 August 2021
27 July 2021
Over the last few years, composer and performer Jennifer Walshe has created a corpus of over 3,500 text scores, ranging from Fluxus performance scores to text scores written by current practitioners. Many of these scores were painstakingly transcribed by Ragnar Árni Ólafsson.
They have been brought together in Text Score Dataset 1.0, to be used as inputs for Machine Learning algorithms which will create new generations of text scores. Commissioned by PRiSM, over the last months Walshe and Ólafsson have been working with David De Roure, PRiSM Technical Director and Professor of e-Research at the University of Oxford, on the first generation of outputs.
The project will be launched at the International Summer Courses in Darmstadt, August 2021 with a presentation of a selection of scores and the launch of a digital booklet of new scores, free to download and perform. We talked to De Roure and Walshe about the project ahead of the launch.
Machine Learning, Language & Sound: The Text Score Dataset 1.0
Project launch with Jennifer Walshe, Ragnar Árni Ólafsson, David De Roure
Live stream Tuesday 3 August 2021 3pm BST (4pm CET, UTC+01:00)
Free tickets available from https://internationales-musikinstitut.de/en/ferienkurse/festival/programm/scoreevent/
Presented every two years by Internationales Musikinstitut Darmstadt (IMD), the Darmstadt Summer Course is an international platform for contemporary and experimental musical practices and composition: summer academy, festival and discourse platform, as well as a meeting point of composers, interpreters, performers, sound artists and scholars.
Interview with Jennifer Walshe
What is a text score?
I’ve been interested in text stores for a long time, since I was a young student and first came across Fluxus (the 1960-70’s art movement) in my classes about John Cage, event scores and experimental music.
A text score is a simple instruction to do an activity (see some examples here). It could as simple as ‘tie a balloon to a piano, pour lighter fluid on it, and set the piano alight’ (Annea Lockwood) or much more complex, for example, what pitches to play, when to play them and in what order. It could be just one word, or pages and pages. In this way, it is very democratic – using just text, not musical annotation, for example.
How did the idea of a dataset of text scores come about?
In 2017, I wanted to make a dataset of pre-existing text scores, then train a machine learning network to create a new body of work. This was the long term goal but first I had to make the dataset. This is a huge task – not just collecting scores but transcribing them, sorting out meta-data, tagging, formatting them. I tried to get as much as I could digitally but asked Ragnar Árni Ólafsson if he could start transcribing them.
Ragnar and I don’t have a background in machine learning, we’re both musicians and interested in composition, so this has been an ongoing 4-year conversation between us. What is relevant to the dataset? What are schools of text scores? What words and fonts pop up again and again?
Also what is lost in the transcription process and digitisation necessary for the data to be read by a machine learning algorithm? As a student I was able to access some original Fluxus scores in Chicago, printed on different types of paper, different textures, holding it is a very different experience to accessing a digitised collection. The conversations elucidated wider issues to do with transcription such as ethics, transparency and bias. What information is being flattened and lost?
The results we want as artists are very different to the results that researchers might want. Ragnar and I are interested in where the indeterminacy of the network offers us interesting juxtapositions – ‘juicy weirdness’ that we can then take into the performance space. A researcher might be looking for something that functions very precisely, very cleanly – something stable and reliable. We are looking for the opposite, we want unreliability.
I asked friends, including James Saunders (co-author with John Lely of Word Events, the definitive book on text scores) with large collections of scores if they could give them to me to pre-train the machine learning networks. I don’t view the text scores created by machine learning as written by me – they are facilitated by me – I am a folklore collector. Ragnar’s role is that of ‘data carer’ – a key role. Nothing was outsourced, he has spent hours and hours transcribing and typing up the text scores to be part of the dataset. A labour of love over a long period of time! As he worked, questions were coming up for him that would then lead to discussions, about language and what a community is. It felt like a chaotic endeavour sometimes, but as the dataset began to take shape, it was really exciting. The dataset is almost an artwork in itself. The physical representation is an artefact.
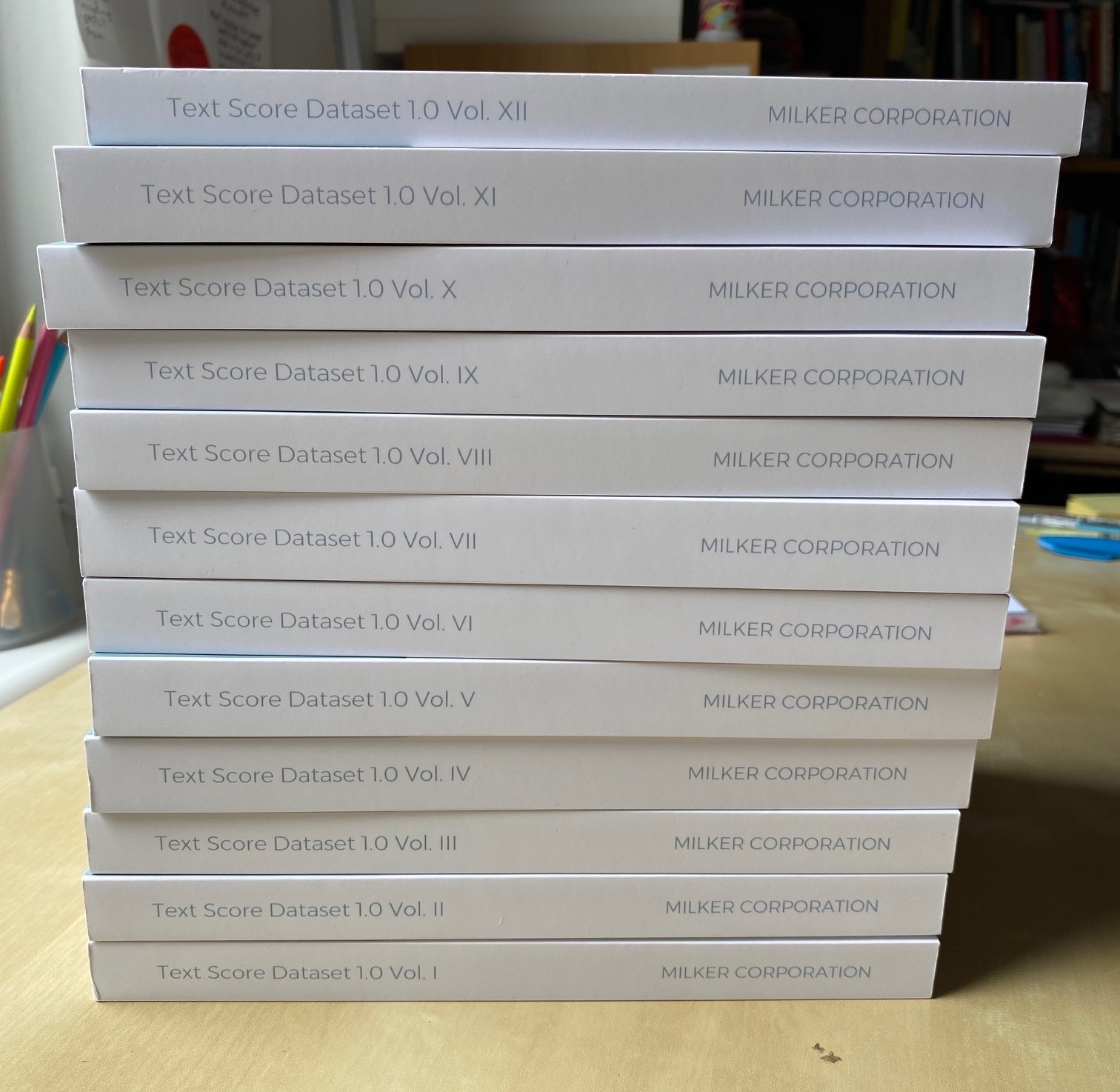
Text Score Dataset 1.0 (image by Jennifer Walshe)
Tell us more about your collaboration with David De Roure
In 2019, I was invited to give a talk at Oxford about AI and my work, including sections from the machine learning project ULTRACHUNK (2018) (Walshe’s collaboration with Memo Akten – watch below and read more here).
We kept in touch and when PRiSM offered a commission, we discussed some areas I wanted to explore. David was very keen to collaborate on text scores and machine learning.
This is the first iteration of this project, which I view as having many more outputs. As part of our work, we’ve been trying different types of neural networks, they aren’t neutral, they have different ‘flavours’, for example, the way that they parse the text. Are you trying to predict text at a word or character level? What is the process of pre-training? All of these contribute to the output. We’re trying lots of different things.
It’s really fantastic to work with someone with David’s background. Our discussions have been stimulating, inspiring and a lot of fun; about machine learning, natural language processing and things like that.
We presented him with the dataset and he started trying different networks. He found ways for us to work directly with the networks, directly intervene, so that we weren’t just handing the data over. We could push it further. There’s a lot of off the shelf tools you can use – we have access to GPT-3 through Open AI, and Turing-NLG through Microsoft – and they are easy to use, but to go much deeper and train on the data set you need someone with David’s skills.
Tell us about the booklet that will be launched on 3 August?
We’re launching a booklet which features a text by myself, a text by Ragnar, and four ‘suites’ of new text scores generated by different neural networks. The booklet also contains ‘varietals’ where the same score sets are fed into different machine learning networks, so that people can see the different results, for example, when you change the weighting or the temperature of the algorithm (parameters of the machine learning network – you can read more about this in the PRiSM blogs A Psychogeography of Latent Space and A Short History of Neural Synthesis). Even feeding the data into the same network can generate different results.
The booklet is digital for now, but we will print it up later in the year. We want people to read the scores, enjoy the cognitive experience, and perform them if they want. There is a plan for a performance of the next generation of scores at a later date. We will also launch a website and ask people to contribute their own scores. For every score contributed, we will generate unique output and give it to them, so crowdsource more scores.
Interview with David De Roure
What attracted you to this project?
The text score corpus – there is nothing else quite like it. Various people in the text score universe have produced text score anthologies, and they are very useful for what we are doing, but this digital corpus is unique.
What is your role in the project?
My role has been to use my knowledge of AI algorithms to adapt networks specifically for text scores. Jennifer and Ragnar had been using off-the-shelf AI models that are publicly available, but I have been able to stick my head under the bonnet and modify the engines. For example, GPT-2 wasn’t trained on text-scores but I was able to fine-tune it with the text score corpus. I can use a lot of text, it’s very fast! So I can generate a lot of outputs, varying the parameters like the level of training and temperature, for Jennifer and Ragnar to review.
The first experiment we did was for me to get the corpus into a state where it could be used to train the AI, but then give Jennifer and Ragnar the ability to change the parameters.
I took one approach – char-rnn which we had initially used on work related to Ada Lovelace in 2018 with Robert Laidlow (Alter, 2018). [Note: the term “char-rnn” is short for “character recurrent neural network”, and is effectively a recurrent neural network trained to predict the next character given a sequence of previous characters. See explanation here https://hjweide.github.io/char-rnn, and a more comprehensive technical explanation here http://karpathy.github.io/2015/05/21/rnn-effectiveness/ ].
By training the AI on the characters individually, rather than a sequence of words, you get the layout, and also you can create new words. So it made a lot of sense to try working at the character level first because text scores are laid out in a certain way. People are playing with the words. Jennifer and Ragnar played with that, and based on their experience I produced the next version.
What have you enjoyed most about the collaboration?
I have done a lot of work with archive corpuses in Digital Humanities – for example the Ada Lovelace correspondence and the Electronic Enlightenment correspondence from a similar period, but this is an entirely different context. I’ve never seen anything like this before – it’s a new kind of corpus and so of great interest to the Digital Humanities community, and on the edge of practice based research.
Interviews by Dr Sam Duffy, July 2021