Sounds from the Funhouse Machine: AI in Composition
18 March 2024
Introduction
The intersection of art and technology has always opened new frontiers for creative exploration, and equally always met with a wary eye. AI is no different, sparking ongoing debates ranging from its influence on human creativity to the question of whose art it is, really.
In this Blog, PRiSM Artist and Producer in Residence Zakiya Leeming traces an exploratory journey of four works created using PRiSM SampleRNN – PRiSM’s first major open-source contribution to the field of machine-learning and audio neural synthesis, likened by the composer to a ‘funhouse machine’.
PRiSM SampleRNN was developed by PRiSM Research Software Engineer Dr Christopher Melen (2019-2023) in collaboration with composer Dr Sam Salem (PRiSM Senior Lecturer in Composition). Launched in June 2020, the development of the software tools was funded by Research England, Expanding Excellence in England (E3).
For background stories of PRiSM SampleRNN:
A Short History of Neural Synthesis | By Christopher Melen
Sounds from the Funhouse Machine: AI in composition
A Four-work Series Exploring PRiSM SampleRNN
By Zakiya Leeming
As a composer who is often informed by science and technology, I was keen to discover what AI-driven tools might offer my practice. I was also curious if I would see a diminished role of the composer or performer in this process. When PRiSM launched its flagship machine learning software PRiSM SampleRNN in 2020, I embarked on a series of works aiming to discover the creative potential of this new tool, whilst contemplating larger questions such as AI’s influence on human creativity, and whose art it is, really?
To understand how I could use the tool, I first needed to understand how it works. PRiSM SampleRNN learns datasets of raw audio by developing statistical predictions about sounds that typically follow a given sample. This is called the model, and it can generate audio based on that understanding. How closely the generated material resembles the original dataset depends on the size of the dataset (more is better), how many times it passed through the dataset (epochs), and a range of other factors including decisions made by the software engineer who helps to run the training processes.
In contrast to some generative models such as ChatGPT, there’s no pre-existing dataset or training. There have been no attempts to teach PRiSM SampleRNN the meaning of ‘music,’ and no supervision in its learning process. This positioned the dataset as the most significant factor under my control. With so many possibilities for how datasets could be curated, I began a series of works that posed different questions about datasets, human/machine relationships, and whether there really was anything new in all of this.
Sad Dog Eating (2021): Homogeneous Beginnings
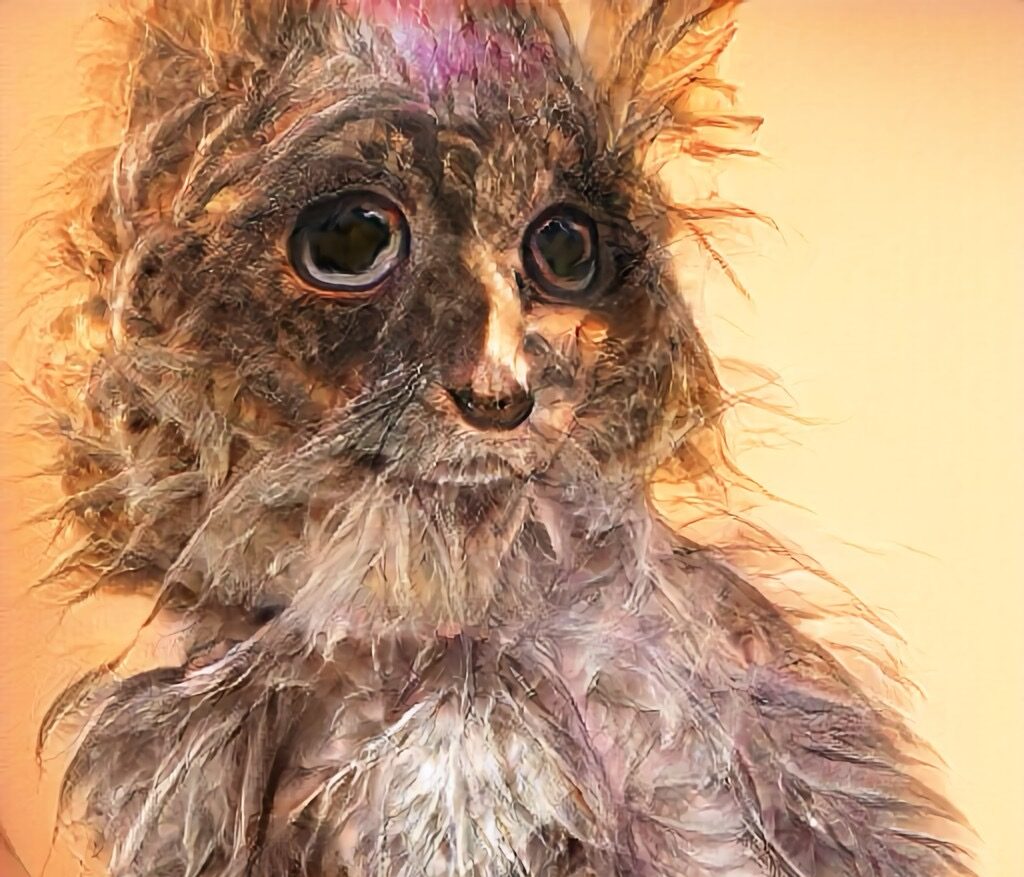
Pictured: Sad Dog Eating (2021) fixed media. Images created with Artbreeder.com, using ’Sad’, ‘Dog’, and ‘Eating’ to create hybrid images and videos.
The first dataset was selected for its homogeneity. Crafted from workshops exploring multiphonics with clarinettists Yanke Dai, Laurel Saunders, and Grace White, the recordings exclusively contained clarinets performing similar sonic material, and any non-performance sounds (such as discussion) was edited out.
The resulting piece was titled Sad Dog Eating, after White’s apt description of a convergence of generated sounds. The work is bookended with the sound of Saunders whistling, with different iterations of the material woven together between, before returning to its identifiably human origin. This includes sounds generated by the machine learning process, sounds produced by the human performers responding to loops created out of that material, and the entire raw dataset itself.
Some generated samples were featured unmodified, while others were cut, layered or otherwise obscured by other sounds. The clarinettists’ response to the machine generated sound, which had in turn been trained on their performances created a spiral of creative reinterpretation that was conceptually interesting, since it is theoretically endless, and because it afforded an ongoing role to the human creators.
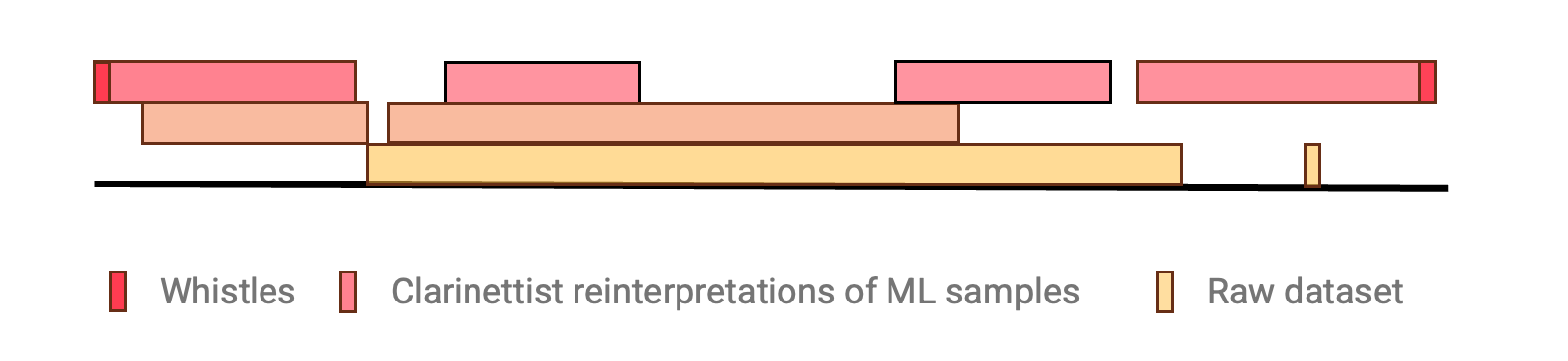
Graphic representation of use of material from three iterations of the process.
I was struck by a question I couldn’t immediately answer – would this process eventually circle the same material endlessly, or would it gradually expose the material factors of the machine learning environment, as a machine-learning cousin of Lucier’s I am Sitting in a Room?
In this first foray into machine learning technology, I was not surprised to find there many creative roles available for humans to take up.
A more surprising realisation was tool felt less like an intelligent machine with the capacity to undermine human creativity, and more like a chamber in which distorted human echoes rang out.
[Intermittent] Cat (2022): Introducing Diversity
The next work was for Solo Tuba & Fixed Media, and the idea behind this dataset was to collect a more diverse set of sounds. Tubist Josh Allen and I had collaborated previously on I Want What They’re Having, a piece exploring distributed creativity together with trio members Keelan Carew (piano) and Jack Sindall (French horn).
During the development of the prior work, we had recorded each of the workshops so that they could be used to train a model for this piece.
In contrast to Sad Dog Eating, the preparation of this dataset did not involve editing non-performance sounds out. Instead, this dataset included a wider variety of audio material, including verbal discussion, preparation to play (blowing through instruments etc.), and interruptions, as well as performance.
From this greater variety in training material, I of course expected the generated samples to follow suit. What I didn’t anticipate what would happen was a non-linear evolution of the generated output at each stage.
When training a model, each pass through the dataset is called an Epoch. During the prior work’s training, the samples I heard progressed more or less continuously toward more accurate representations of material in the dataset.
But the generated samples from different Epochs of [Intermittent] Cat had distinctly different characteristics.
I attributed the difference to two factors: the greater variety of sounds in the new dataset, and the earliness at which samples were generated.
The samples used for Sad Dog Eating were taken from Epochs ranging in the 100s – 300s. Material used in the tape track for [Intermittent] Cat ranged only from Epochs 2 – 60.
This irregular progression of material started with different granularities of noise, developed to chattering speech-like sounds, with a sudden swing to what I called the ‘learning moaning tuba’ Epoch and through to different types of instrumental and speech sounds. This informed the structure of the work as a steady but non-linear transformation of sound, each with its own distinct persona.
A Bluetooth speaker was suspended in the bell of the Tuba, allowing the machine generated material in the tape part to be played through the instrument, alongside the live performance. This reflected origin of the recorded material: the trio. The concept was to integrate the three instruments together in a physical space (the Tuba) to mirror a lack of differentiation in origin of sound that resulted from the training and audio generation process. Allen and I then worked together to find sounds that audibly interacted with the material being emitted from the speaker and through the instrument, such as the interaction of water in the Tuba with the static sounds in the tape and air being blown through the mouthpiece.
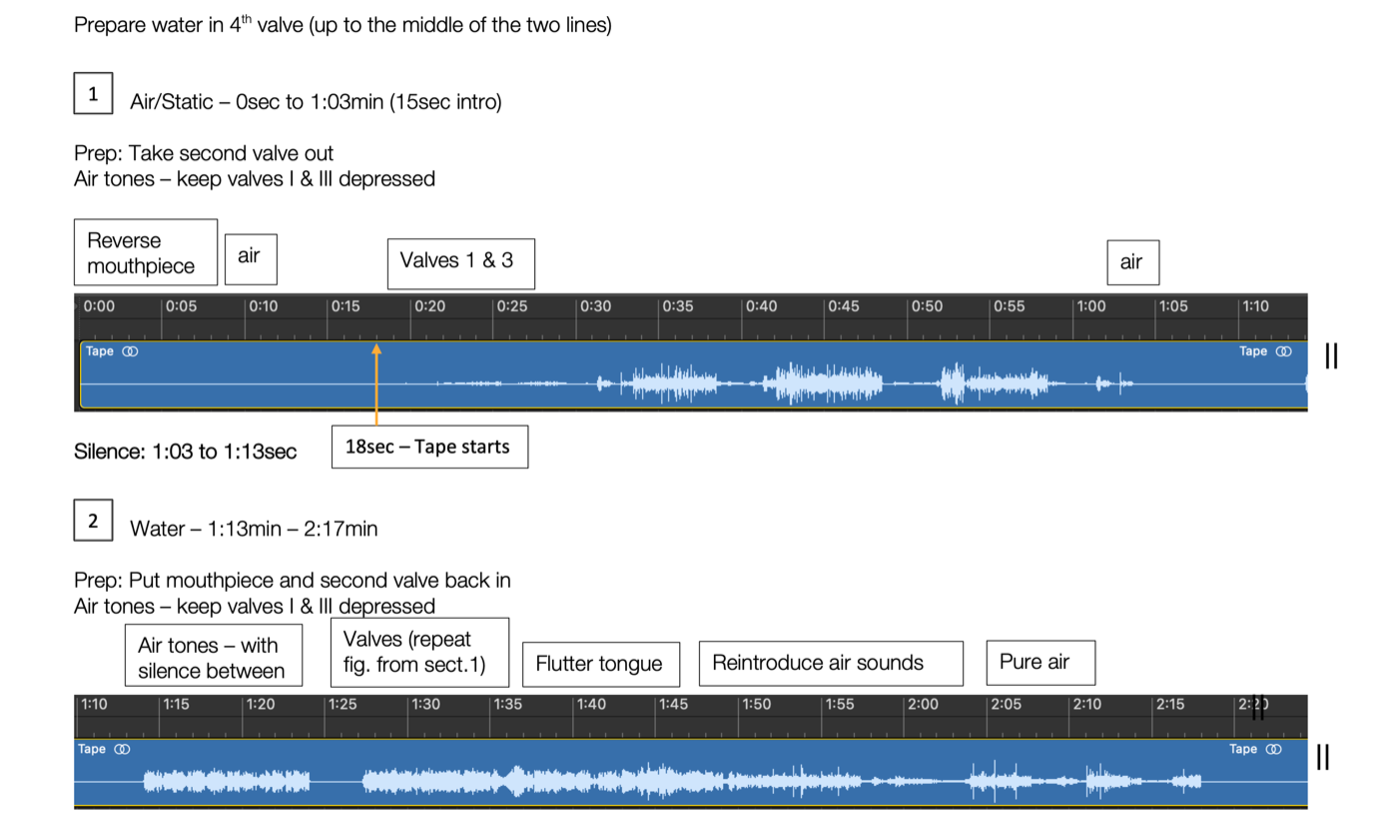
[Intermittent] Cat (2022): Score Excerpt
Sometimes. In Dreams. I Still. (2023): The Temporal Challenge
So far, I had created one model for each ensemble. I was curious whether distinct outcomes would emerge if the same ensemble responded to different scores, and different models were created for each. I created three scores for the Riot Ensemble to test this idea.
Splitting recording time between three scores meant I was working at the limits of dataset size. Since I didn’t want to risk altering the weighting of the original material through data augmentation – the practice of deriving more data from a small dataset – using pitch shifting, frequency banding, time stretching etc., I decided instead to merge the recordings from Dataset Scores I and III into a single model, against the markedly different material of Dataset Score II.
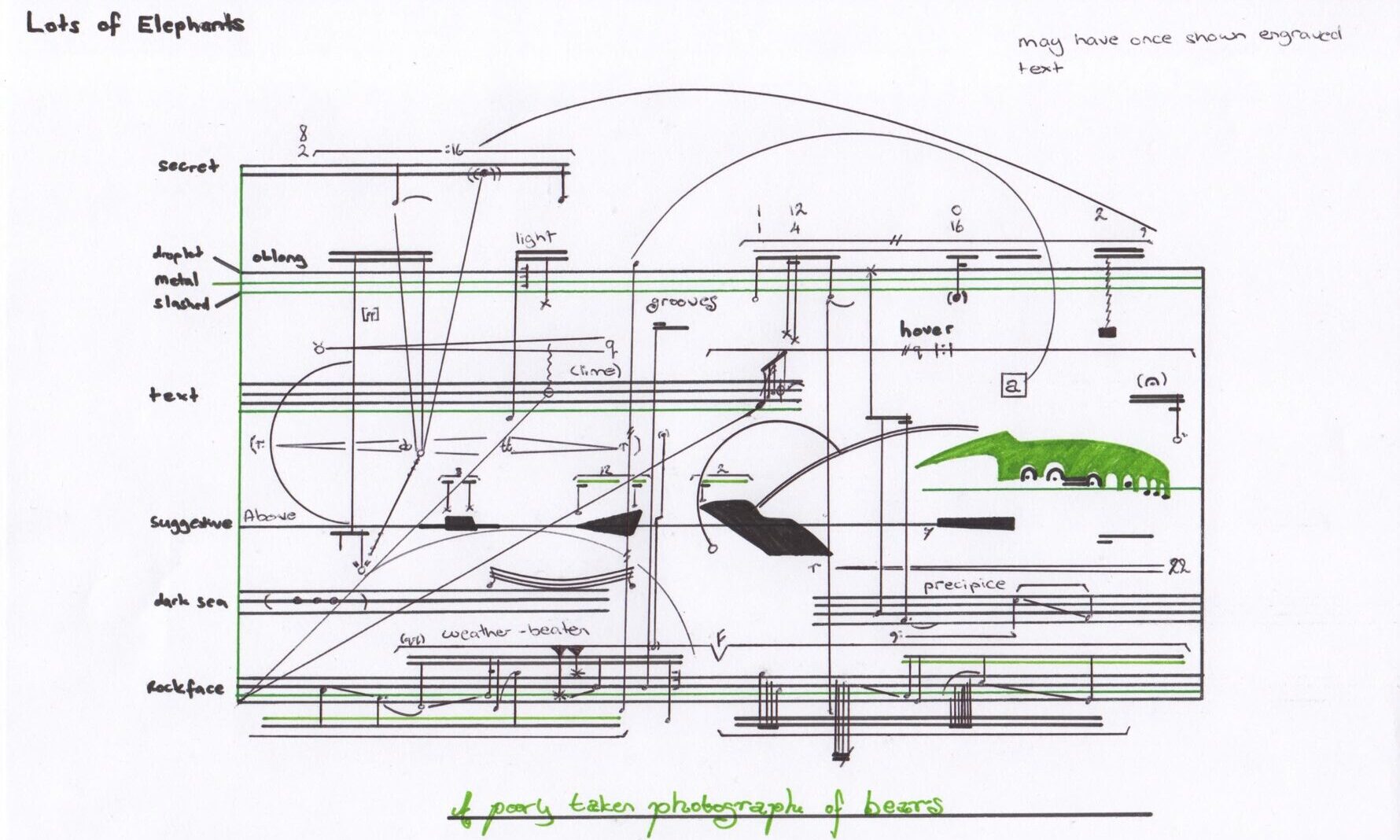
Dataset Score I: The First: A poorly taken photograph of bears
Score II, used to train the second model, was an attempt to create a ‘meta-instrument’. Here, every sound in the dataset was a variation of same iambic rhythm, played by different combinations of at least two performers.
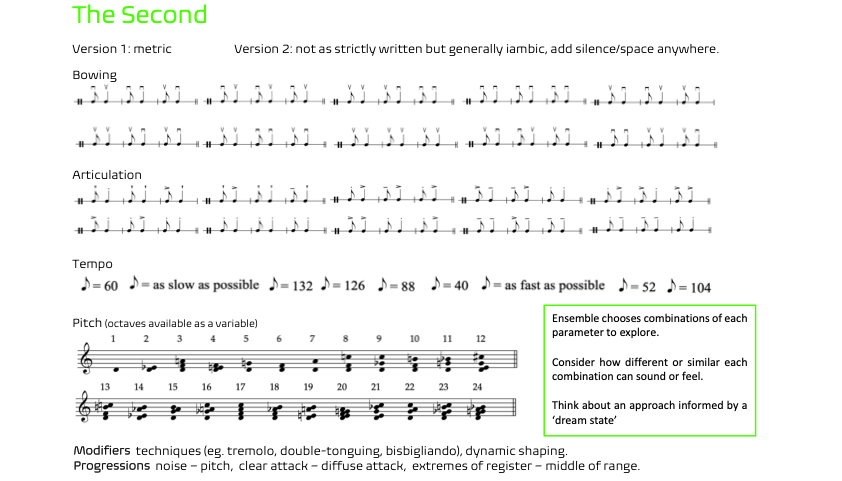
Dataset Score II: The Second. Experiments in rhythm and meta-instruments.
The generated samples from the two resulting models did result in different qualities of sound. Whilst Score II successfully created sounds that were audibly derived from more than one instrument, they did not always achieve sufficient blend to be considered a ‘meta-instrument’ throughout.
Sometimes. In Dreams. I Still. used the distinct qualities of each of the sets of generated samples to differentiate the two main sections of the work.
The initial composed material is intended to blend with the dry popping, clicking and scraping material of the tape track, such that the distinction between the ensemble and generated material is obscured, and the ensemble is amplified to aid this.
The second section takes the relative grittiness of Score II as a basis for the live performance material:
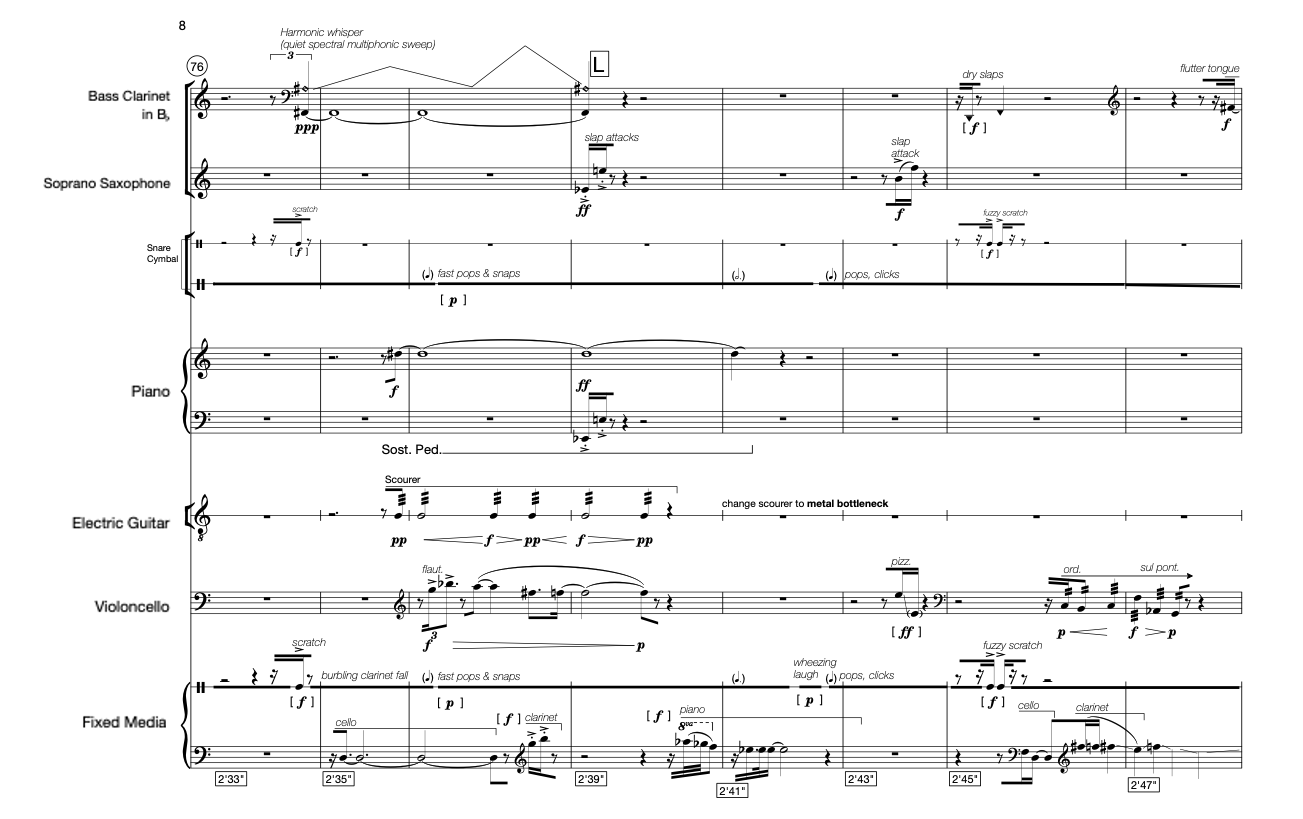
Sometimes. In Dreams. I Still. (2023): Score Excerpt
Here the idea of the ‘meta-instrument’ is widened to include the live version of the ensemble with the versions of itself in the fixed media.
Both become progressively distorted as if in a feedback cycle that ends in the engulfment of the live ensemble by the generated and processed version of itself.
Working with small datasets and specific end results in mind, such as the construction of a meta-instrument, this idea pushed the use of PRiSM SampleRNN to certain limits.
As expected, a rhythm featured in all material in Score II did not result in its appearance in the generated material, confirming that temporal and structural elements were unlikely to be learned by a sample-by-sample prediction model.
But its use in creating a meta-instrument does have further potential with differently curated datasets.
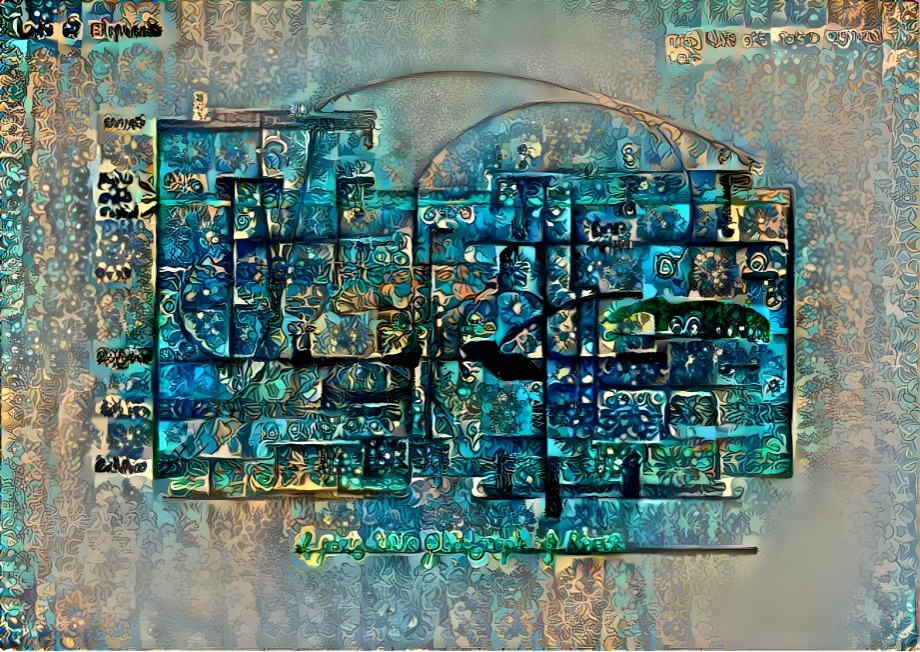
Dataset Score III: The Third: Interpretation 3.
Meanwhile. In Other Dreams… (2023): The Influence of AI on Creative Outcomes
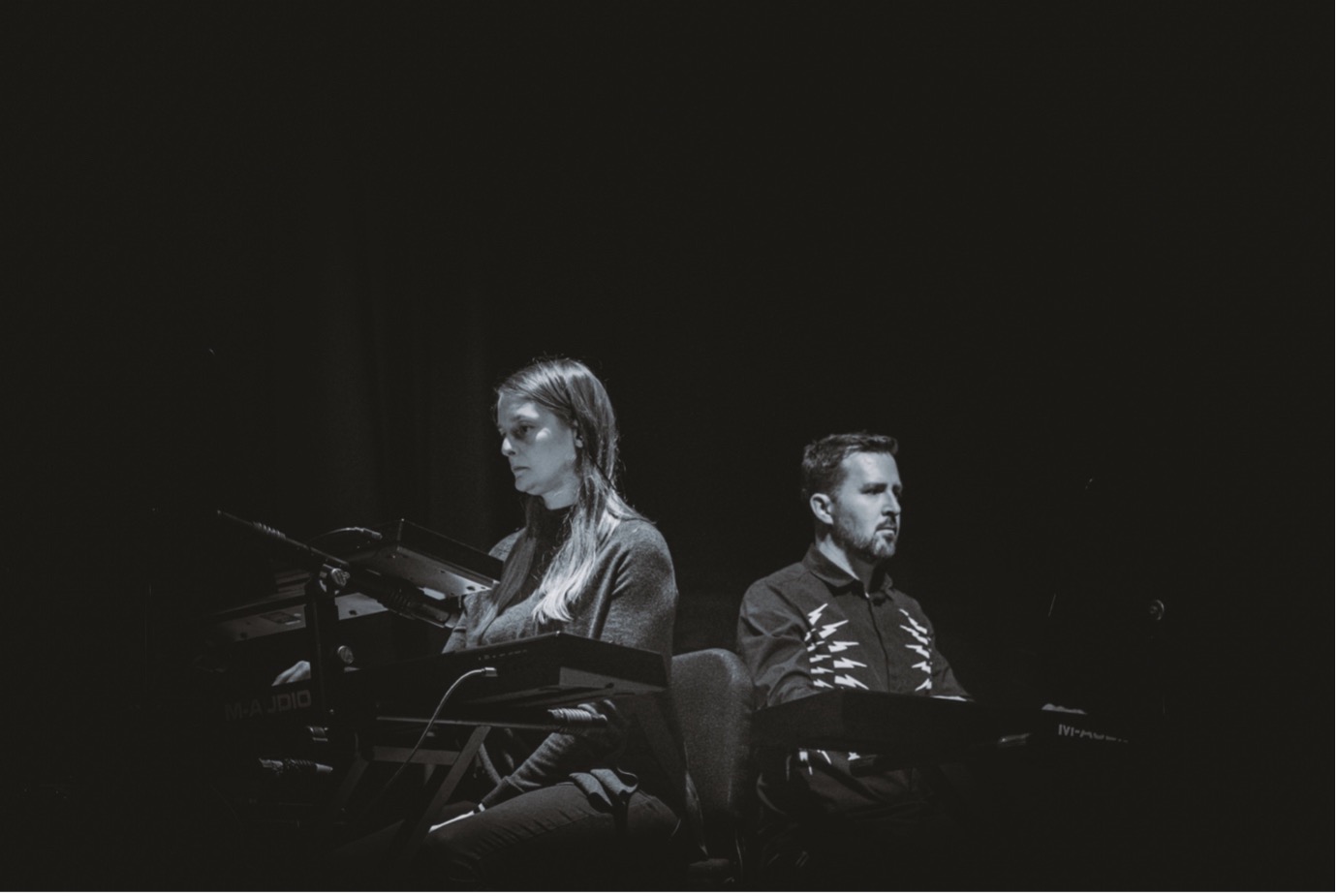
Riot Ensemble keyboardists Adam Swayne & Siwan Rhys performing ‘Meanwhile. In Other Dreams…’
‘Meanwhile…’ was written as a companion piece to ‘Sometimes…’, as both were written for the Riot Ensemble and developed from the same generated material.
In this work, I wanted to confront what I perceived as a recurring fallacy surrounding AI music – the notion that it is somehow solely driven by computers, detached from human influence.
Above all, the previous works in this series had highlighted to me not only the ongoing role of the human performers in a machine learning process, but the necessity of their presence for the existence of the process in the first place.
This work brought the two back together, the performer and the machine-generated responses, but with the tables turned. The keyboard players are triggering the generated samples programmed into their instruments, placing control of the machine-generated sound derived from their performance back under their fingers.
Conclusions: the Unique place of PRiSM SampleRNN
This four-work series allowed me to explore the challenges and limitations of this tool, understanding the versatility within those parameters while developing a sense of its unique potential and materiality of sound. I discovered useful and interesting processes involving AI-based tools; the opportunity to engage in a dynamic process between performers, scores, datasets, programmers, myself and back again, and the ability to create potentially endless iterative cycles of creativity, each with their own unique set of contributing people and factors.
I avoided pursuing realistic likenesses of datasets in the generated samples, opting at times for something closer to glitch aesthetics, demonstrating the potential to employ machine learning tools for ‘off label’ purposes.
During the time in which I worked with this software, many more machine-learning tools have become available for use with sound and music. Some offer deeper learning, faster results, improved structural and temporal frameworks, and appear to be closer to the powerful, impressive tools long promised (and feared). But spending an extended time experimenting with the parameters of a single, now more comparatively rudimentary tool allowed me to ask questions both of the basic mechanisms whilst they’re still possible to comprehend, and also of the place of machine-learning within my creative practice.
My question about the reduction of the human role in the creative cycle proved not misjudged as such, but rather a misunderstanding of how the technology worked within a larger artistic apparatus and context. There was never not a human present. Not in the original data that the software was trained on, nor in the decision-making process that created the methodology and the selection of sounds, and not least in the programming of the tool itself. If anything, this experience magnified the presence of the human at every juncture.
Since it turned out there was a human behind each curtain, the question ‘whose art is it, really?’ turned out to have no meaningful difference to the one I had been asking in other collaborative spaces, and with different creative tools built with digital technology.
That said, the ability to press a single button and instantly generate the next ‘contemporary classical 10min work for crunchy gutteral strings’ may very well be nearly here, and the place, meaning and response to such tools will become the next terrain ripe for artistic interrogation.
But despite the rapid evolution of machine learning technology, I have found value in steadily exploring the unique materiality and uses of PRiSM SampleRNN. If machine learning can only reflect back to us what we put in, tools that can now convincingly recreate human performance could be viewed as a hall of mirrors. But there remains a special place in my creative toolkit for the funhouse mirror that is PRiSM SampleRNN.